Mastering Success: The Impact of NFL Analytics on Teams’ Competitive Edge
Introduction: The Rise of Analytics in the NFL
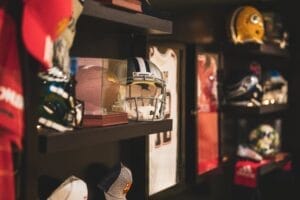
In recent years, the use of analytics in the NFL has become increasingly prevalent. Teams are now relying on data-driven decision making to gain a competitive edge in a league that is constantly evolving. The importance of analytics in today’s game cannot be overstated, as teams are using advanced metrics and technology to improve scouting, game strategy, player evaluation, and in-game decision making.
This article will explore the evolution of data analytics in football, how teams are utilizing analytics to improve various aspects of the game, the role of advanced metrics in player evaluation and drafting, the impact of analytics on in-game decision making, how teams are leveraging data to improve player performance and injury prevention, the use of machine learning and AI in football analytics, the challenges and limitations of analytics in the NFL, case studies of successful teams embracing analytics, and predictions for the future of analytics in the NFL.
The Evolution of Data Analytics in Football
The use of analytics in football is not a new concept. In fact, it has been around for decades. However, with advancements in technology and the availability of more data, the use of analytics has become more sophisticated and widespread. In the early days of football analytics, teams relied on basic statistics such as yards gained and touchdowns scored to evaluate players and make strategic decisions. As technology improved, teams began to collect more detailed data such as completion percentage, yards per attempt, and time of possession. This allowed teams to gain a better understanding of player performance and make more informed decisions.
Today, teams have access to an unprecedented amount of data thanks to advancements in technology. With the use of GPS tracking devices, teams can collect data on player movement, speed, acceleration, and distance covered during games and practices. This data can then be analyzed to identify patterns and trends that can inform game strategy and player evaluation. Additionally, teams are now using video analysis software to break down game film and identify strengths and weaknesses in both their own team and their opponents. This allows teams to make more informed decisions on play calling and game planning.
From Scouting to Game Strategy: How Teams are Utilizing Analytics
One of the key ways that teams are utilizing analytics is in the area of scouting and player evaluation. In the past, scouts relied on their own observations and subjective opinions to evaluate players. While this approach can still be valuable, teams are now using analytics to supplement their scouting efforts. By analyzing data such as college statistics, combine results, and player performance in specific situations, teams can gain a more objective view of a player’s abilities and potential.
For example, teams may use advanced metrics such as yards per route run or quarterback rating under pressure to evaluate wide receivers or quarterbacks, respectively. These metrics provide a more nuanced view of a player’s performance and can help teams identify players who may be undervalued or overlooked by traditional scouting methods. Additionally, teams are now using analytics to identify specific traits or skills that are important for success in their particular system. This allows teams to target players who possess these traits and increase the likelihood of success.
In addition to scouting, teams are also using analytics to shape game strategy and play calling. By analyzing data on opponent tendencies, formations, and play outcomes, teams can identify patterns and trends that can inform their game plan. For example, if a team notices that an opponent tends to run the ball on third down in short yardage situations, they may adjust their defensive strategy accordingly. Similarly, if a team notices that a certain play has been successful in similar situations in the past, they may be more likely to call that play in a similar situation in the future.
The Role of Advanced Metrics in Player Evaluation and Drafting
Advanced metrics play a crucial role in player evaluation and drafting decisions. These metrics provide a more comprehensive view of a player’s performance and can help teams identify players who may be undervalued or overlooked by traditional scouting methods. One example of an advanced metric that is commonly used in player evaluation is Pro Football Focus (PFF) grades. PFF grades players on every play of every game, taking into account factors such as technique, execution, and impact on the outcome of the play. These grades provide a more objective view of a player’s performance and can help teams identify players who may be flying under the radar.
Another example of an advanced metric that is commonly used in player evaluation is SPARQ (Speed, Power, Agility, Reaction, and Quickness) score. SPARQ is a composite score that takes into account a player’s performance in various athletic tests such as the 40-yard dash, vertical jump, and shuttle run. This score provides a measure of a player’s overall athleticism and can help teams identify players who may have the physical tools to succeed at the next level.
Teams are also using advanced metrics to inform their drafting decisions. By analyzing data on college statistics, combine results, and player performance in specific situations, teams can gain a more objective view of a player’s abilities and potential. For example, teams may use metrics such as yards per route run or quarterback rating under pressure to evaluate wide receivers or quarterbacks, respectively. These metrics provide a more nuanced view of a player’s performance and can help teams identify players who may be undervalued or overlooked by traditional scouting methods.
The Impact of Analytics on In-game Decision Making
Analytics have had a significant impact on in-game decision making in the NFL. Teams are now using data to optimize play calling and game management, with the goal of maximizing their chances of success. One area where analytics have had a particularly big impact is in fourth down decision making. In the past, coaches would often rely on gut instinct or conventional wisdom when deciding whether to go for it on fourth down or punt the ball away. However, analytics have shown that teams are often better off going for it on fourth down, especially in certain situations.
For example, analytics have shown that teams are often better off going for it on fourth down when they are in opponent territory and need less than five yards for a first down. In these situations, the potential reward of converting the fourth down and keeping the drive alive outweighs the risk of not converting and giving the opponent good field position. By using analytics to inform their decision making, teams can increase their chances of success and potentially gain a competitive advantage.
Another area where analytics have had an impact is in play calling. By analyzing data on opponent tendencies, formations, and play outcomes, teams can identify patterns and trends that can inform their play calling. For example, if a team notices that an opponent tends to blitz on third down in long yardage situations, they may be more likely to call a screen pass or draw play to take advantage of the aggressive defense. Similarly, if a team notices that a certain play has been successful in similar situations in the past, they may be more likely to call that play in a similar situation in the future.
How Teams are Leveraging Data to Improve Player Performance and Injury Prevention
In addition to scouting and game strategy, teams are also using analytics to improve player performance and prevent injuries. By monitoring player performance data such as speed, acceleration, and distance covered during games and practices, teams can identify areas where players may be underperforming or at risk of injury. This allows teams to make adjustments to training programs or recovery protocols to optimize player performance and reduce the risk of injury.
For example, if a player’s speed or acceleration numbers are consistently below average, teams may work with the player to improve their conditioning or technique. Similarly, if a player’s workload is consistently high and they are at risk of overuse injuries, teams may adjust their practice schedule or workload to reduce the risk of injury. By using data to monitor player performance and make informed decisions, teams can optimize player performance and reduce the risk of injury.
Teams are also using analytics to optimize training and recovery programs. By analyzing data on player workload, sleep patterns, and recovery metrics, teams can identify areas where players may be at risk of overtraining or underrecovering. This allows teams to make adjustments to training programs or recovery protocols to optimize player performance and reduce the risk of injury.
For example, if a player’s workload is consistently high and they are at risk of overuse injuries, teams may adjust their practice schedule or workload to reduce the risk of injury. Similarly, if a player’s sleep patterns are consistently poor and they are not recovering adequately, teams may work with the player to improve their sleep habits or recovery protocols. By using data to monitor player performance and make informed decisions, teams can optimize player performance and reduce the risk of injury.
The Use of Machine Learning and AI in Football Analytics
Machine learning and AI are playing an increasingly important role in football analytics. These technologies are being used to analyze large amounts of data and identify patterns and trends that may not be immediately apparent to human analysts. By using machine learning algorithms, teams can uncover insights that can inform decision making and improve performance analysis.
One area where machine learning and AI are being used is in opponent scouting. By analyzing large amounts of game film and play data, machine learning algorithms can identify opponent tendencies, formations, and play calls. This allows teams to gain a better understanding of their opponents’ strengths and weaknesses and develop game plans that exploit these weaknesses.
Another area where machine learning and AI are being used is in player evaluation. By analyzing large amounts of data on college statistics, combine results, and player performance in specific situations, machine learning algorithms can identify patterns and trends that may not be immediately apparent to human analysts. This allows teams to gain a more objective view of a player’s abilities and potential and make more informed decisions on drafting and player acquisition.
The Challenges and Limitations of Analytics in the NFL
While analytics have become increasingly prevalent in the NFL, there are still challenges and limitations to their use. One challenge is the availability and quality of data. While teams now have access to an unprecedented amount of data, not all data is created equal. Some data may be incomplete or inaccurate, which can lead to flawed analysis and decision making. Additionally, not all data is publicly available, which can limit the ability of teams to make informed decisions.
Another challenge is the interpretation and application of data. While analytics can provide valuable insights, it is up to teams to interpret and apply this data in a meaningful way. This requires a deep understanding of the game and the ability to translate data into actionable strategies. Additionally, there is always a risk of overreliance on analytics and neglecting other important factors such as coaching expertise, player intangibles, and situational context.
Case Studies: Successful Teams Embracing Analytics
Several successful teams in the NFL have embraced analytics and used data-driven decision making to gain a competitive advantage. One example is the New England Patriots, who have consistently been one of the most successful teams in the league over the past two decades. The Patriots have been known for their meticulous attention to detail and their use of analytics to inform game strategy and player evaluation. They have used advanced metrics such as yards per route run and quarterback rating under pressure to identify undervalued players who fit their system.
Another example is the Kansas City Chiefs, who won Super Bowl LIV in 2020. The Chiefs have embraced analytics in all aspects of their organization, from scouting to game strategy to player performance analysis. They have used data to identify opponent tendencies and develop game plans that exploit these tendencies. They have also used analytics to monitor player performance and prevent injuries, which has helped them keep their key players healthy and performing at a high level.
The Future of Analytics in the NFL: What to Expect in the Coming Years
The future of analytics in the NFL is bright, with continued advancements in technology and the availability of more data. In the coming years, we can expect to see even more sophisticated use of analytics in all aspects of the game. Teams will continue to refine their use of advanced metrics and machine learning algorithms to gain a competitive edge. Additionally, we can expect to see more integration of analytics into coaching and player development, as teams look for ways to optimize performance and reduce the risk of injury.
One area where we may see significant growth is in the use of wearable technology. As technology continues to improve, teams will have access to even more detailed data on player performance and health. This data can then be used to inform training programs, recovery protocols, and injury prevention strategies. Additionally, we may see advancements in virtual reality and augmented reality technology, which could revolutionize how teams analyze game film and develop game plans.
In conclusion, the use of analytics in the NFL has become increasingly prevalent in recent years. Teams are now relying on data-driven decision making to gain a competitive edge in a league that is constantly evolving. The evolution of data analytics in football has been driven by advancements in technology and the availability of more data. Teams are utilizing analytics to improve scouting, game strategy, player evaluation, and in-game decision making.
Advanced metrics play a crucial role in player evaluation and drafting decisions, while machine learning and AI are being used to analyze large amounts of data and uncover insights that can inform decision making. While there are challenges and limitations to the use of analytics in the NFL, several successful teams have embraced analytics and used data-driven decision making to gain a competitive advantage. The future of analytics in the NFL is bright, with continued advancements in technology and the availability of more data.